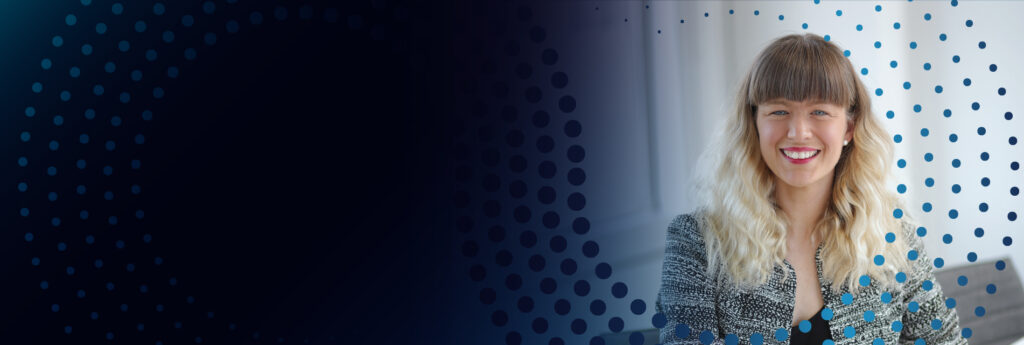
It seems that algorithmic underwriting (AU) is taking the insurance industry by storm; but while mainstream products such as house and car insurance are seeing AU being put to good use, specialty insurance is relatively new to the game. Part of the reason is down to data.
As a form of computerised automated decision-making, AU relies on data to follow a set of instructions, the most basic of which is “if X happens, carry out this action; if Y happens, carry out that action”. Unlike artificial intelligence (AI) – which can adapt instructions depending on the data or results of a sequence – AU doesn’t have this level of machine learning, so if you put unusable data into the system, your results will be unusable too.
To enable AU to work successfully, therefore, insurers need data that is cleansed and correctly formatted. They also need the right infrastructure, such as cloud-based services, plus the right underwriting knowledge and technological know-how, points of which have been highlighted by a leading Insurtech report.2
The importance of ecosystems
The first step to deliver suitable data for AU is data cleansing and standardisation, a process which can be both time-consuming and prone to errors. This is especially true for specialty lines of insurance and risk analysis, where data cleansing can involve multiple manual tasks, such as geocoding risks, mapping columns and data conversion to feed downstream systems. Not only is this process slow, but it has to be carried out for individual clients and MGAs on an annual / monthly basis.
For insurers to harness the true benefits of AU, they need system(s) that can automate these tasks and make data machine-usable, from automating geocoding and providing audit trails, to identifying new lines of insurance and even simply storing information.[1] Implementing such processes tends to work best when done in tandem with other systems and technologies, a feat which can be achieved through strategic partnerships with subject matter experts.
The best partnerships are those where the insurer can focus on what they are good at (i.e. underwriting), while the external experts help in the areas that they know best (i.e. from a technology perspective).
Taking the next step
There is an abundance of data currently in insurance, and with new innovations around sharing data and streamlining processes, such as AU, it is becoming even more vital that data is cleansed properly, is in a consistent format – and above all, actually usable to get results.
As the saying goes, garbage in = garbage out. Applying advanced tools like AU to the underwriting process will not lead to good results if the underlying data is unformatted, unstructured and uncleansed. Increasingly we are seeing insurers turn to advanced new augmented intelligence tools to help them get to grips with these data fundamentals – tools that learn to sweep through their data schedules and find, fix and structure data before they flow through to the next stage. With clean data, insurers can then successfully use AU, allowing them to quote faster and estimate claims quicker, without re-cleansing the data on a regular basis.
Deploying clean data is half the battle when using AU; the other half is being able to bring the right talent and skills on board to actually build the frameworks needed to deliver such automated underwriting decisions. Because at the end of the day, it is all about good data, not data for data’s sake. With clean data, underwriters can not only apply these new technologies properly, but they can have a better grasp on their portfolio, meaning they can write better quality business with confidence and apply the most accurate pricing models.
———-
[1] Algorithmic underwriting in commercial and specialty insurance
Published with the kind permission of Fintech & Finance News
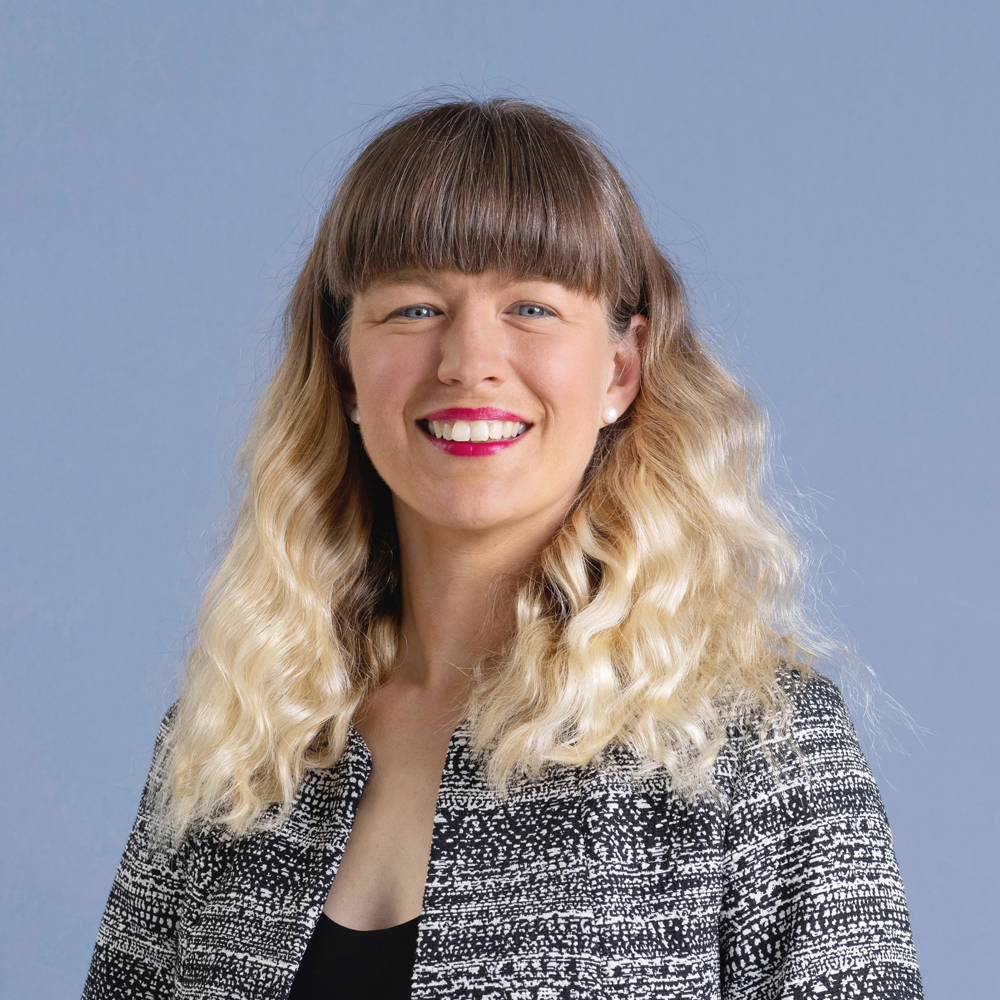
Meet our expert
Name: Louisa Pavis
Job title: Head of Consulting
Get in touch
To speak to the Pro Global team please feel free to reach out to us at:
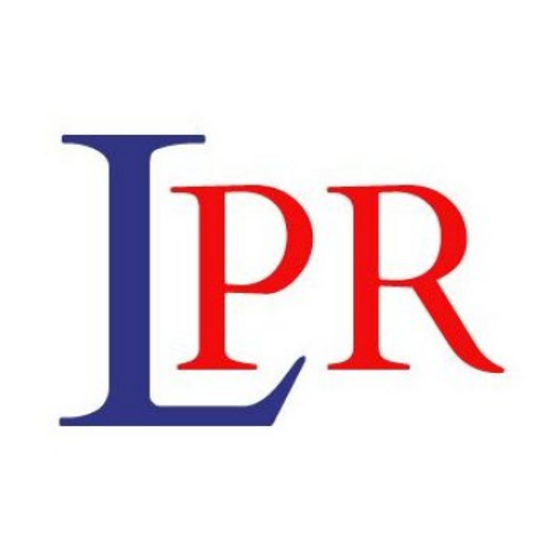
Lysander PR
To contact our PR team directly please use the link below